What is Machine Learning (ML)?
What is Machine Learning?
Machine learning (ML) is a field devoted to understanding and creating methods and algorithms that leverage data to “learn” and streamline computing tasks. As a sub-field of Artificial Intelligence, machine learning algorithms use “training data” to build predictive models to make decisions without the need for programming. These algorithms enable computers to learn on their own.
A fundamental assumption that informs machine learning algorithms is that strategies, algorithms, and inferences that have worked well in the past will likely work well in the future. These observations intend to make likely predictions and can be obvious, like predicting the lifespan of a specific species, or nuanced, like expecting a certain medical condition in one group because it is present in a similar group. When you feed a machine learning algorithm data, there are tasks the algorithm can perform without any need for programming. Human-created algorithms have limitations in that a human has to explicitly outline all the exact steps needed to complete the task. With machine learning algorithms, more complex workflows can be developed by enabling the machine to program its own algorithms.
Machine learning algorithms also tend to refine data to approach answers. For example, suppose you ask a question, and there is a vast possibility of solutions. In that case, the algorithm can identify all the potential answers to create a smaller subset of data and repeatedly run tasks on it to widdle the data set towards a likely conclusion.
Historically the field of machine learning emerged out of the quest to develop artificial intelligence. The field evolved in the 1990s to focus more on practical problem-solving routed in mathematical models, namely statistics, fuzzy logic, and probability theory. The primary difference between artificial intelligence and machine learning is that machine learning makes predictions and decisions based on observations. In contrast, artificial intelligence implies environmental interaction to learn and make decisions. Another related field, data mining, differs from machine learning in that it focuses on discovering properties within a data set rather than making predictions.
How Machine Learning is Used Today?
Machine learning algorithms are used across most industry sectors, ranging from agriculture to medicine to entertainment to computer security. Let’s look at some examples of how machine learning is used today in everyday applications:
- Google Maps – Google can predict the fastest directional routes by feeding all current location and movement data from its users into an ML algorithm.
- Social Media – apps like Facebook use ML algorithms to detect faces in photos and suggest friends you should tag.
- Ridesharing – apps like Uber use your travel history and behavior patterns to suggest locations you likely want to travel to.
- Access Control – organizations are leveraging software that uses ML algorithms to automate the levels of access an employee should have by analyzing job profiles and other data.
- Wildlife Preservation – organizations use ML algorithms to track and analyze endangered species populations and movements to enhance conservation efforts.
- Healthcare Applications – Healthcare providers are leveraging ML algorithms for a number of use cases, for example, to predict waiting times and manage staffing.
These are just a few examples of the thousands of ML algorithms used across numerous industries.
Machine Learning & Identity Management
Using analytics, artificial intelligence (AI), and machine learning (ML) to improve enterprise identity security is critical to outpace cybersecurity threats. Rather than buzzwords, leaders want to see real-world use cases where human and machine intelligence meaningfully converge.
We expect more in-depth use of analytics and AI/ML technologies to improve risk awareness and decision-making for identity-related business processes. While loftier benefits – like totally human-less access decisions – are some years away, others are accessible now.
Saviynt & Machine Learning
Innovations are irrelevant if they don’t produce the outcomes enterprises expect. Identity platforms often promise – but don’t deliver – lower risk profiles, improved decision-making, reduced compliance violations, and hardened security postures built around Zero Trust. Saviynt is shifting the narrative and transforming IGA and PAM with robust AI/ML and analytics built into our Identity Cloud. Here are some breakthroughs we’re delivering now.
Advanced Analytics
As organizations shift to the cloud, agile delivery models or ineffective Identity Governance may lead to excessive access. Sharing the ‘keys to the kingdom’ can be disastrous; few applications are designed with least-privilege principles, and advanced analytics are required to reduce emerging risks.
Saviynt uses data-rich analytics to simplify identity intelligence. Armed with insights, identity analysts and administrators can identify and mitigate risks accurately and rapidly.
For example, organizations can streamline IAM Policy creation and create a consistent definition of identity and access based on user attributes. Analytics-driven attribute-based access controls (ABAC) and role-based access controls (RBAC) help enterprises better manage least privilege access. With focused analytics, enterprises can narrow definitions of ‘access’ and control what end-users can do – while reducing Separation of Duties violations.

Saviynt uses AI & ML to determine identity risk and offer smart review/approval.
Deloitte recognizes the use of behavioral analytics to create baseline markers of normal user behaviors. Additionally, built-in natural language processing (NLP) can help easily monitor for abnormal occurrences – and learn (and infer) from behavior patterns. This supports faster, frictionless identity-related decision-making.
During our recent CONVERGE 20 Roadshow, Michael Allred, Director of Identity and Access Management at Intermountain Healthcare, shared how his team benefits from analytics (and from making smarter, more secure decisions) at scale: “Reviewing user-behavior and auditing access for 50,000 users is simply not sustainable.”
With Saviynt’s actionable analytics, enterprises can quickly visualize data and risks and respond promptly. These include real-time insights into user risk changes due to excessive access or the presence of activities outside a role/user’s typical behavior. Insights lead to smarter controls, too, including creating and managing access policies & roles.
Intelligence without actionability isn’t useful, which is why Saviynt integrates with (and ingests data from) SIEM and UEBA solutions. Most companies use multiple tools to evaluate their security postures, which requires managing large quantities of logs, alerts, and notifications. Our platform intelligently curates these – adding visibility and risk scoring to support anomalous activity detection and incident responses.
AI & ML
Central to ‘intelligent identity’ is smarter work underpinned by AI & ML. One of the most useful applications of these technologies is risk modeling and decision-making.
For example, we recently announced Assisted AI, which bridges human and machine intelligence interactions to create better governance strategies. Identity-centric security starts with knowing who accesses what resources, why they need access, and how they ultimately use that access.

Saviynt’s Risk Insight Panel assesses risk from different sources and aids data-driven decision making.
As access approvals and denials occur, Saviynt gathers data from each request made. Our platform curates and learns from the data; eventually, the AI precisely understands appropriate responses to common request scenarios. This alleviates the burden of repetitive requests and enables approvers to focus on unusual or high-risk requests.
Our automation also reduces costs associated with birthright provisioning, continuous monitoring, and governance. We’re excited about how automated dynamic provisioning leads companies toward zero standing privilege and just-in-time access principles. Along the way, we’re creating linkages between all identifiers, cataloging and discovering assets, and making time-series observations of these relationships to build recommendations and personalization.
Organizations utilizing Saviynt have seen up to 36% of SoD violations prevented during the access request process. This reduction translates to managers saving time when reviewing excess access. Historical data, platform analytics, and peer benchmarks are feeding our AI to help drive actionable authorization decisions, as well.
Our AI & ML recommendation engine supports other insights, including business rule and policy suggestions, and recommendations to improve role quality, ownership, and user membership. Saviynt’s work to build a fully automated, self-learning platform is part of our mission to transform IGA and create a truly “prescriptive” identity experience.
Cynicism around AI/ML and advanced analytics in the identity market is often warranted. At Saviynt, we don’t just market a smarter platform — we deliver one.
Risk reduction, security improvements, centralized access control, increased productivity, cost reduction, and regulatory compliance assurance are direct results of infusing intelligent functionality into our Identity Cloud platform.
We’re proud of our performance to date. But the use cases above – and recent breakthroughs like Identity BOT (built-in RPA engine to automate provisioning) and Identity Exploration (helping users explore trends and determine patterns in identity data) – are just the beginning.
Resources
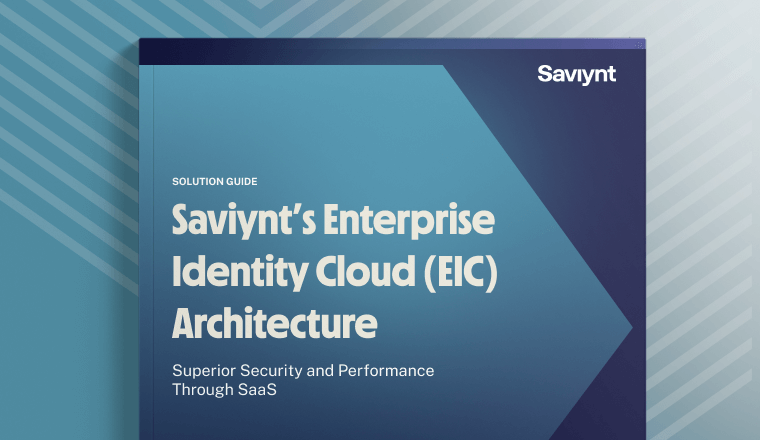
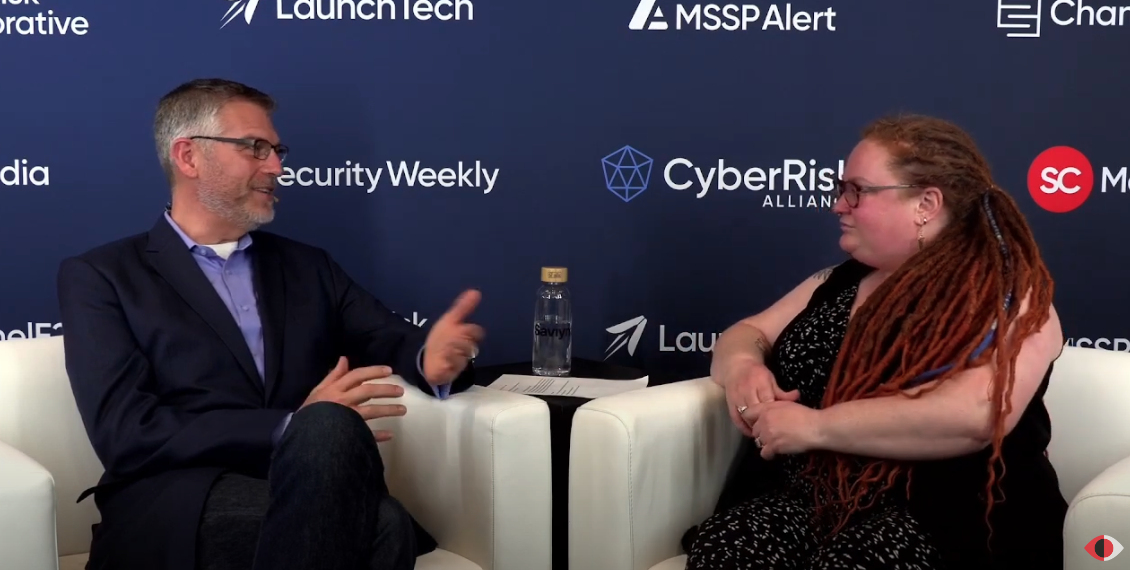
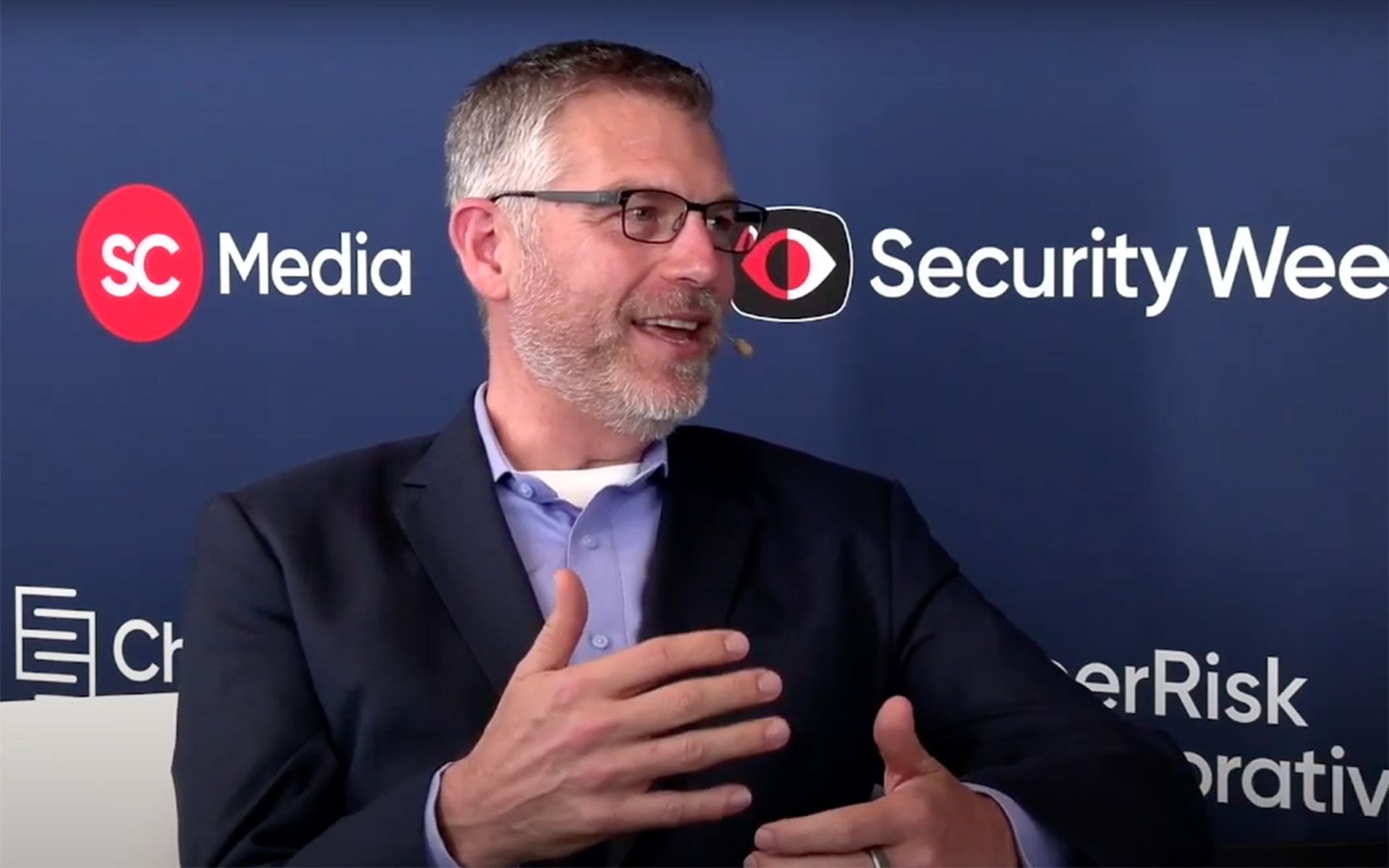